Cancer-Net SCa could offer dermatologists a low-resource tool to improve skin cancer screening
New software developed with the help of artificial intelligence (AI) could support dermatologists to detect cancerous skin lesions earlier and more accurately.
University of Waterloo Professor Dr. Alexander Wong led a team of researchers that developed Cancer-Net SCa, a suite of deep-learning AI algorithms designed to identify skin cancer from dermoscopy images.
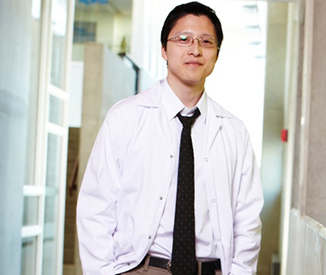
“Our goal was to give dermatologists more information so they can more accurately distinguish cancerous lesions,” says Wong, an OICR Affiliate and Canada Research Chair in Artificial Intelligence and Medical Imaging.
Skin cancer is the most frequently diagnosed cancer, and detecting it early is critical to successfully treating it. Screening with a dermotoscope – a device that illuminates and magnifies lesions – is an increasingly common approach for early detection.
But the visual differences between malignant and benign lesions are subtle, and even experienced dermatologists can have a hard time distinguishing between them in a dermotoscope image.
“Visual assessment is really tough,” says Wong. “Malignant lesions can easily be mistaken for something benign and vice versa.”
Wong and colleagues wanted to create a tool that dermatologists around the world could integrate into their practice. That meant building something fast and accurate that didn’t require huge amounts of computing and storage.
With such specific requirements, they couldn’t just adapt an existing neural network – they had to build one from scratch. But that would be extremely time-consuming if done by hand. Instead, Wong and colleagues leveraged another AI program to build their AI for them, providing the specifications they needed and allowing the machine to determine the best architecture to meet them.
“Our unique AI-building-AI approach helped us create a neural network that balanced our performance requirements with our efficiency requirements,” Wong said.
Cancer-Net performed well in testing, as reported in a recent BMC Medical Imaging paper, delivering fast results with about 84 per cent accuracy. But Wong says accuracy numbers only tell half the story – it’s just as important to understand why the algorithms classified a lesion as benign or malignant. Wong and colleagues therefore designed Cancer-Net to provide explanations for its decisions.
These explanations were used to validate Cancer-Net’s effectiveness, understand why it sometimes made inaccurate decisions and ultimately to help improve its performance. Wong also thinks these explanations will be crucial to their software’s clinical utility, helping to build dermatologists’ trust.
“If doctors don’t understand why AI is making a decision, they’re not going to use it,” Wong says.
Researchers are now working to collect more data and build better models, with new iterations of Cancer-Net on the horizon. They have also made their algorithms open source to encourage researchers and clinicians to use them and build upon them.
“We hope that these low-resource models can integrate very well into existing clinical decision systems, and act as a sort of ‘virtual assistant’,” Wong says. “Hopefully this gives dermatologists insights that assist them in coming to a well-informed decision.”