New proof-of-concept approach offers faster, more accurate inferences about tumour populations.
The prevalence of mutations in a tumour can provide clues about how the cancer has grown or evolved and how best to treat it.
But making predictions about the evolution of mutated tumours using a single DNA sequenced biopsy requires complex estimates, and current methods are either slow, or don’t always consider why certain subpopulations of cells proliferate more than others.
This led a pair of researchers at the Ontario Institute for Cancer Research (OICR) to develop a new method for understanding and predicting cancer evolution that harnesses modern deep learning algorithms and incorporates evolutionary modeling.
TumE is a deep learning approach that analyzes the frequency of mutations in a biopsy — known as the variant allele frequency — to identify which subpopulation of mutated cells that are most “fit” and thus most likely to make a tumour stronger.
“We’re taking deep learning tools into the cancer genomic space and trying to find optimal methods for predicting and understanding cancer evolution,” says lead researcher Tom Ouellette, a PhD student at OICR based in Dr. Philip Awadalla’s lab.
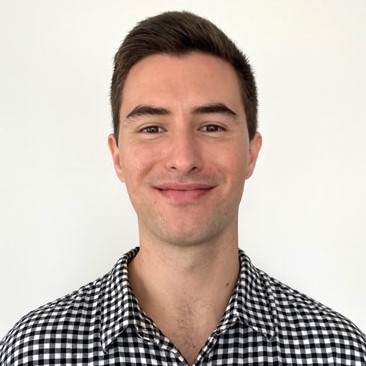
TumE’s algorithm uses evolutionary modeling to distinguish between cases where highly “fit” cellular subpopulations are driving tumour growth versus cases where no one cell or subpopulation has a detectable advantage. This helps it make predictions about how many subpopulations are observed in a tumour biopsy, and which subpopulations and mutations will eventually take over.
Understanding which mutations will increase in frequency can provide insight for therapeutic intervention or for quantifying the aggressiveness of tumour growth.
TumE builds on two other methods for inferring the evolution of mutations — approximate Bayesian computation and mixture models — which have been used for several years but have limitations. When tested with simulated data and 88 whole genome sequence samples, as reported in PLOS Computational Biology, TumE generated inferences that were faster than approximate Bayesian computation and more accurate than mixture models.
Though it’s still at the proof-of-concept stage, Ouellette says that TumE offers a good foundation for quickly understanding the link between mutations and the evolutionary dynamics that drive cancer. With access to more data, he says the approach could be scaled up to make more complex predictions.
“With high-quality data and with minimal computational cost, we could use methods like TumE to find patterns and start making statistical estimates of which mutations lead to worse outcomes,” he says.
The advantage of deep learning is that, once something like TumE has been developed, it can be re-tuned to predict different things without having to start from scratch. Eventually, Ouellette says deep learning could be used to determine which mutations or subpopulation of cells are most deadly and should be targeted with precision cancer treatments, ultimately leading to better outcomes for patients.
“We’re trying to find methods that can be used quickly and concurrently with patient care,” he says. “I think this is a stepping stone to get there.”
TumE is publicly available for use at https://github.com/tomouellette/TumE