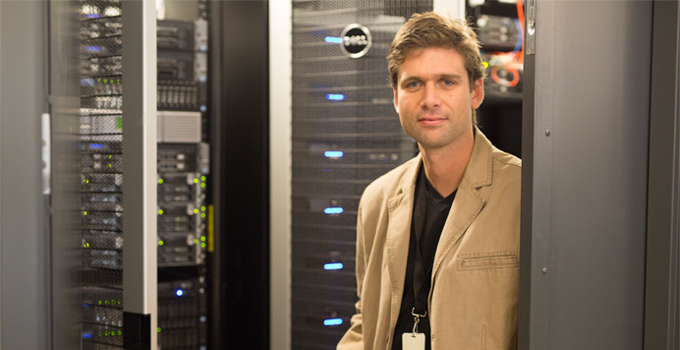
Meta-analysis of 1,200 patients with pancreatic cancer reveals a new way to identify those with very aggressive tumours who may benefit from alternate treatment approaches
Only half of pancreatic cancer patients who undergo standard chemotherapy and surgery live a year after their initial diagnosis. In the face of these dismal statistics, patients are faced with the challenge of deciding whether they want to proceed with treatment that may have unpleasant side effects. If clinicians could identify patients who would not benefit from standard therapies, they could help these patients make more informed treatment decisions or recommend alternative palliative treatment approaches.
As part of OICR’s Pancreatic Cancer Translational Research Initiative (PanCuRx) team led by Dr. Steven Gallinger, Dr. Benjamin Haibe-Kains recognized that computational modeling can be used to help inform these decisions, but to design a robust predictive model he would need much more data than any individual study had ever collected.
Building the data foundations
Haibe-Kains, who is a Senior Scientist at the Princess Margaret Cancer Centre and OICR Associate, began his investigation with a dataset from PanCuRx – the largest collection of genomic and transcriptomic data on primary and metastatic pancreatic tumours to date. He and his lab then incorporated an additional 1,000 cases of pancreatic tumours from studies around the world that had collected both patient samples and information about how each patient responded to treatment.
“The datasets that we aggregated were a mixed bag of different types of data collected through different profiling platforms by different institutions,” says Haibe-Kains. “We took on the challenge of harmonizing the heterogeneity of these resources which nobody else had done.”
Previously, the Haibe-Kains Lab developed a computational method that could make incompatible transcriptomic data compatible. They had used this method to find four new breast cancer biomarkers to predict treatment response and they recognized that they could apply similar methods to harmonize pancreatic cancer data as well.
The dataset resulting from the harmonization is now the largest pancreatic cancer dataset, and Haibe-Kains has made it freely available for other researchers to use and study through the MetaGxPancreas package.
Making a predictive model
Haibe-Kains and his team set out to develop a computational model that could predict if a patient would survive for a year after their biopsy. They used machine learning techniques to exploit their rich dataset, find common patterns in the genomic data of aggressive tumours, and developed PCOSP – the Pancreatic Cancer Overall Survival Predictor.
“Our approach was to look at how one gene was expressed relative to another and relate that to how long a patient lived after biopsy,” says Haibe-Kains. “That may sound simple, but that means dealing with nearly 200 million pairs of genes, which is a significant amount of data to compute.”
As recently described in JCO Clinical Cancer Informatics, the group refined PCOSP using ensemble learning – the combination of several machine learning techniques to improve a model’s accuracy of predictions.
“PCOSP is actually a combination of hundreds of models and not just one,” says Haibe-Kains. “We tested about a thousand models, selected the models that could predict early death very well and combined them to make a stronger classifier.”
Using prediction to power patient decisions
Haibe-Kains says that as the infrastructure for routine sequencing progresses, PCOSP can be translated into clinical practice to help clinicians determine which patients would not benefit from standard treatment and which may benefit from alternative treatment approaches.
“Pancreatic cancer is a challenging disease but if we can predict the course of the disease, we can give clinicians and patients more information. With that information, they can make more personalized decisions to improve their treatment and ideally, their lives.”