Research led by OICR and SickKids used machine learning to find links between cancer outcomes and druggable proteins.
Ontario scientists used advanced computing techniques to generate a catalogue of potential targets for future drug discovery research, as well as two promising targets for brain cancer drugs.
In a study published in the EMBO Journal, researchers used machine learning — a type of artificial intelligence (AI) that can learn from data and make predictions — to analyze the gene expression of more than 9,000 tumour samples across 33 cancer types.
They looked specifically at a group of proteins called “ion channels”, which have been targeted by drugs for cardiovascular disease but have been understudied for cancer therapeutics. By looking at the expression of these ion channels in the tumour samples, the machine learning algorithm identified about 400 potential targets that linked to patient survival in one of 33 cancers.
“These proteins may have a role in how cancers grow, and the catalogue we developed could be a useful resource for researchers looking to develop or repurpose drugs for cancer,” says OICR Investigator Dr. Jüri Reimand, whose lab led this research alongside Dr. Xi Huang’s lab at SickKids.
From this catalogue, Reimand and colleagues noticed that four proteins seemed particularly linked to patient outcomes in glioblastoma, the most common and deadliest brain cancer. The team at SickKids then did experiments on two of these proteins in their brain cancer research lab. They discovered that the two ion channels help control how rapidly glioblastoma cells grow, and that when the proteins are disabled in brain cancer cells the tumours become less aggressive in mouse models. One of the ion channels also appears to control cell-cell communication networks of brain cancer cells.
“That opens the door for further work developing brain cancer therapeutics and also adds to our understanding of how brain cancer cells work,” says Reimand, who is also Associate Professor in the Department of Molecular Genetics at the University of Toronto.
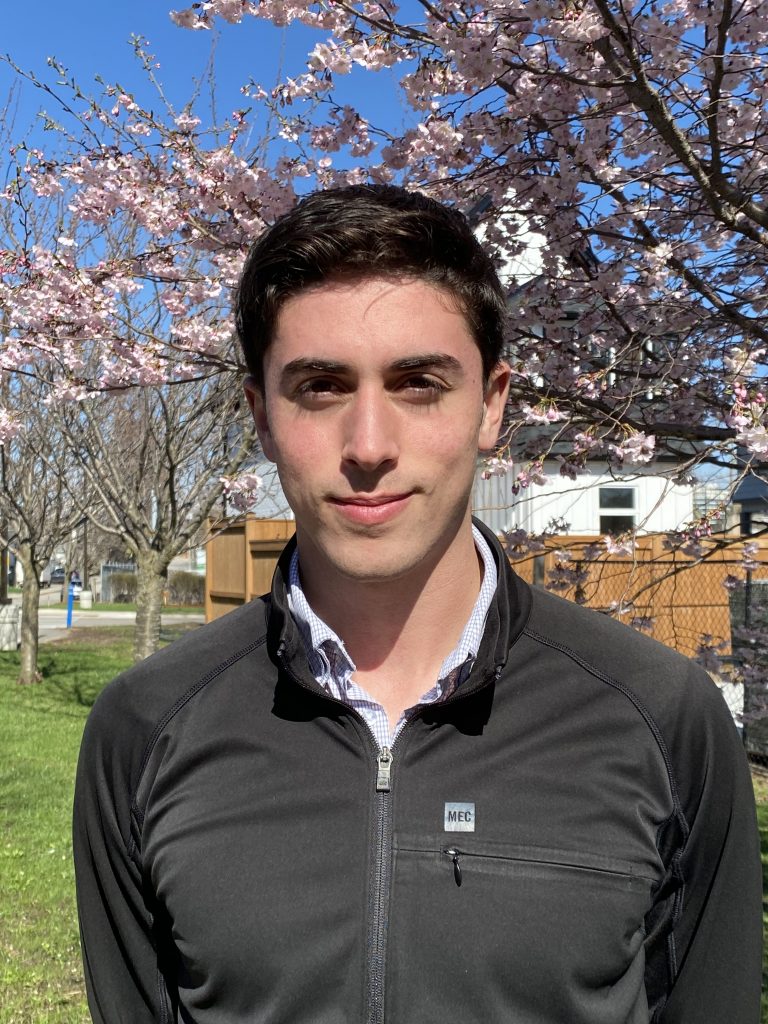
The study is the culmination of long-term collaboration between Reimand and Huang that began when they were both postdoctoral researchers. This latest work was led by PhD students Alexander Bahcheli and Nicolaes Hyun-Kee Min, who are members of the Reimand and Huang labs and PhD students at the Department of Molecular Genetics at the University of Toronto.
“It’s exciting to explore these different classes of proteins from a cancer perspective,” says Bahcheli, who completed this research as part of his PhD. “Machine learning allows us to find the strongest associations that may be most relevant to future research.”
Machine learning has shown promise in drug discovery research because of its ability to parse through huge amount of data relatively quickly. With machine learning, the teams at OICR and SickKids were able to focus in on two promising targets without having to run hundreds of experiments to identify and validate targets, which could take years to complete.