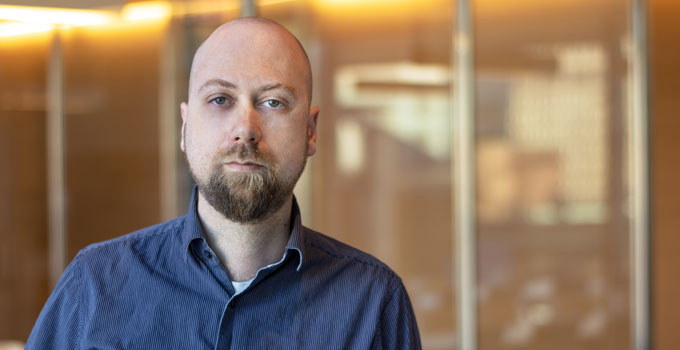
The tool can accurately distinguish real mutations from sequencing mistakes to improve the early detection of cancer
DNA mutations in cancer cells are caused by different processes, each of which leaves a genetic fingerprint that can provide clues to how the cancer develops. Researchers have now applied this understanding to reduce errors when reading DNA, allowing them to accurately and efficiently detect the smallest traces of mutated cells in the blood.
In a recent publication in Science Advances, an OICR-supported research group outlines a new and improved statistical model to reduce error rates in DNA sequencing data. They demonstrate that their model, called Espresso, outperforms current error suppression methods.
“When we isolate, amplify and try to read the individual building blocks of DNA, we encounter a lot of errors,” says Dr. Sagi Abelson, OICR Investigator, Assistant Professor at the University of Toronto and first author of the publication. “This is a major obstacle. The high error background makes it difficult to pinpoint authentic rare mutations. This is what Espresso aims to solve.”
To build an effective error-suppressing statistical model, the group assessed the different types of errors in their relative genomic contexts across more than 1,000 sequencing samples. Their approach was based on assessing the genetic fingerprints within these samples and mapping them to the regions around the errors to understand if the error was a true mistake, or if it was an important mutation.
“The key advantage of our method is that it allows scientists to read DNA more accurately without the need to duplicate efforts using a set of independent control measurements to estimate error rates,” says Abelson. “This means that researchers can be more efficient with their time and resources. They can do more with less. We’re proud to have developed methods that can make research more practical and simple, but also more effective, efficient and accurate.”
This model is built on Abelson’s prior research published in Nature, which discovered early indicators of acute myeloid leukemia (AML) in the blood up to 10 years before symptoms surfaced. With Espresso, the research group was able to develop and test a new strategy to predict leukemia development, which could predict up to 30 per cent of AML cases years before clinical diagnosis with extremely high specificity. Importantly, this study demonstrated that the risk of developing AML can be measured by looking into only a small number of genomic bases, which suggests a more practical route to clinical testing and implementation.
“This work builds on our prior research, which has shown that we can detect AML earlier than thought possible,” says Dr. John Dick, Senior Scientist at the Princess Margaret Cancer Centre, Co-lead of OICR’s Acute Leukemia Translational Research Initiative and co-senior author of the study. “With these methods, we’ve now shown that we can focus in on specific areas of DNA to detect those early traces of AML with higher accuracy than ever before.”
“These methods are essential to advancing personalized cancer care in practice,” says Dr. Scott Bratman, Senior Scientist at the University Health Network’s Princess Margaret Cancer Centre and co-senior author of the study. “With these tools, we can enable clinicians to treat cancer more effectively, tailor treatment decisions and monitor minimal residual disease. We look forward to furthering our research for patients today and those who will develop cancer in the future.”